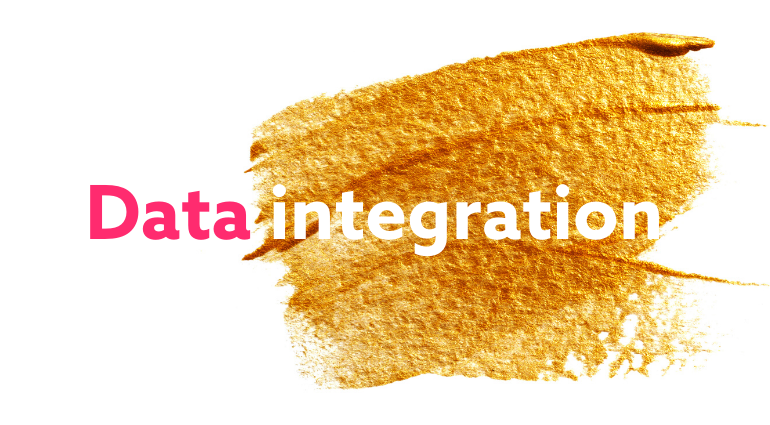
Data is a valuable asset for telecommunication companies. But only processing the data makes its true potential evident – when large data volumes are turned into knowledge. This cannot be done without data integration: It creates added value from data.
Table of contents
- Data flood and data integration
- Use cases for data integration with telecommunication companies
- Challenges
- Conclusion: Much work, and even more benefits
1. Data flood and data integration
Today, nobody can ignore the power of data: Companies are data-driven, decisions are made based on data, in some countries even a person’s social status depends on data. It goes without saying that not all data is relevant for all applications. Whether artificial intelligence, predictive maintenance or our old friend the weather forecast: Every application needs the appropriate data to work properly.
This is where data integration comes into play: Data integration is understood to mean merging of data from several different sources in pursuit of a specific purpose, usually with the aim of using the data meaningfully. Data integration goes beyond the mere collection and clear-up of the data (e.g. erasing duplicate data): Also mapping (establishing relationships between data models) and transformation (transfer of the data from its original structure to a different one) of data is an integral part of the process.
The benefit of this concept is evident: If, for example, a company launching a troubleshooting tool defines which data is relevant for this use case, this data can be automatically supplied and analyzed permanently. This means that relevant data is constantly fed to the tool. Based on this data, it can draw conclusions, suggest actions, and ultimately rectify faults.
Data flood
The following practical example illustrates the principle: The Dataflood family have a problem with their diaries. Dad John manages his appointments in the calendar of his email application. Mum Jane enters her appointments in the calendar which hangs at the kitchen wall. Daughter Jenny uses the calendar app in her smartphone. To check whether her family is free to visit Granny for coffee and cake on Sunday, Mum Jane needs to ask every family member separately. This takes time and is definitely inconvenient. In short: The Datafloods have a data integration problem.
The solution is a uniform family calendar which can access all the relevant data sources, in this case the calendar in Dad’s email program, Mum’s kitchen wall calendar and Jenny’s calendar app. When introducing the family calendar, the family defines these data sources to allow for integration of the relevant data. Then, a tool can automatically set the different data in relation to each other.
Data integration & digital transformation
Wherever and whenever applications are based on data, data integration takes place in the background. Robust data processing is mandatory because digital transformation makes data more complex, bigger, and more important. To put it simply: The more appointments the members of the Dataflood family have, the more important it is to keep an overview. Or: The more important data is becoming, the more important is clean data integration. This is widely proved by examples from the telecommunication industry.
2. Use Cases for data integration with telecommunication companies
SIMBOX fraud detection
The fight against criminals is a major topic in the field of telecommunication. One example is the SIMbox fraud where cheaters forward phone calls via a SIMbox and the Internet to avoid the fees for international calls. The characteristics of these illegal phone calls are slightly different from those of legal calls.
The relevant data is for example the date, time and duration of a call, the phone numbers, and information on the country, region, identity, account, and customer segment. Mobile calls forwarded via a stationary SIMbox are often directed through the same base station. With legal phone calls, on the contrary, more frequent changes of the base station occur. Combining several of these findings, the companies have a better chance of discovering frauds.
Customers ready for a smartphone change
For telecommunication companies, one of the goals of predictive analysis is to know when a customer will be ready to change his or her smartphone. A company which detects this user behavior soon enough will be able to orchestrate the change – for example by making the customer an individually tailored special offer.
To this end, Telcos create profiles based on historic data and allow the provider to recognize the conditions leading to a customer’s decision to get a new phone. Comparing these profiles with current data, the provider can identify customers who may be ready for a phone change. This use case requires the input of various data – from the users’ income bracket up to the average emotional brand loyalty.
Troubleshooting of high-speed Internet connections
Also a troubleshooting tool needs access to data which affect for example the functionality of a high-speed Internet connection. Here, particularly the OSS (network management systems or service delivery platforms) and BSS (CRM, contracts database or sales software) are relevant. With the analysis of this data, error sources can be identified and eliminated, for example by comparing snapshots. What is particularly challenging here is the involvement of a large number of data suppliers.
3. Challenges
Clean data integration is not at all a matter of course. Many people tend to forget one aspect: The major precondition for identifying relevant data sources is understanding the data. Having a vast volume of useful data will not help you at all if nobody knows the significance and correlations of the data. Judging from the present state of the art, the quality of the data content should always be assessed by humans.
There are other challenges which are not evident when defining relevant data sources but come to light after the start of the application. A classical example are the so-called releases:
The surrounding systems which are the source of the relevant data are not static but subject to constant further development. In the course of these releases, the data supplier will regularly modify its interfaces, for example by adding new data fields. The data consumers must react to these changes: They must ensure that they will get what they need for their specific application. Accordingly, the data supplier should communicate any modifications in data type or interfaces so that the data consumers can accommodate for them. Unannounced modifications will result in faulty or incomplete data – and thus often in total failure.
Five other challenges may impede data integration, as can be seen from the example of the Dataflood family:
- Poor data quality: Obsolete or non-updated data records are a major problem: Important information is missing in this case. Sometimes, external data is not as detailed as internal data, or does not come up to the requirements.
For the Dataflood family, poor-quality data would for example be appointments that have been canceled but not yet erased in the family members’ calendars .
- Data silos: Often, data records are hoarded in so-called data silos instead of being shared with others. If the respective data is relevant for a certain application, the execution of this application will be poor or even impossible.
If a member of the family kept an appointment secret from the others, he or she would create a kind of data silo.
- New data formats: At some point in time after the initial definition of the data sources, new data formats are added. The tool must be able to integrate and transform the new formats.
If Mum Jane decides to use a pocket diary instead of her wall calendar, the uniform family calendar must be able to process this new data format.
- Currentness: Changes to data integration projects are not limited to the data level. The projects also need to adjust to new situations (such as new requirements of the company).
Granny Dataflood has fallen ill and moves in with the family. Now, her calendar must be integrated in the family calendar as well.
- Data volume: Particularly large use cases which are affected by lots of different data are particularly challenging. The more data sources there are, the more complex their integration.
Unifying the calendars of a family of three is not too difficult – unifying the calendars of all employees of a company is a much more demanding task.
4. Conclusion: Much work, and even more benefits
Data integration is much initial work and is not a one-off task. It involves permanent care, monitoring and updating of the data and the connected structures. Data integration is a basic prerequisite for the mere functionality of an application – and thus ultimately for the functioning of business models and companies. The high initial effort will be reduced in the foreseeable future: Automated data analysis and transformation tools will produce relief here.
Data integration: Creating added value
Nothing goes without data – it is the prerequisite of any type of applications. However, the true potential of data only becomes evident once the data is combined. Companies combine different data sources, deduce findings, and take measures on this sound basis. Taken on its own, a single data record will only tell you if an Internet connection works or not. After a troubleshooting tool has combined several data sources, however, it becomes possible to identify the cause and predict the pattern of a malfunction. This combination of data is made possible by data integration, which thus creates added value from the data.
Our Dataflood family clearly demonstrates the added value: The original reason for combining their data was Granny’s invitation for Sunday afternoon. Short-term benefit: coffee and cake. The long-term benefit of unifying everybody’s calendars, however, is much more valuable: more harmony and better coordination among the members of the Dataflood family. How about this for a happy ending?